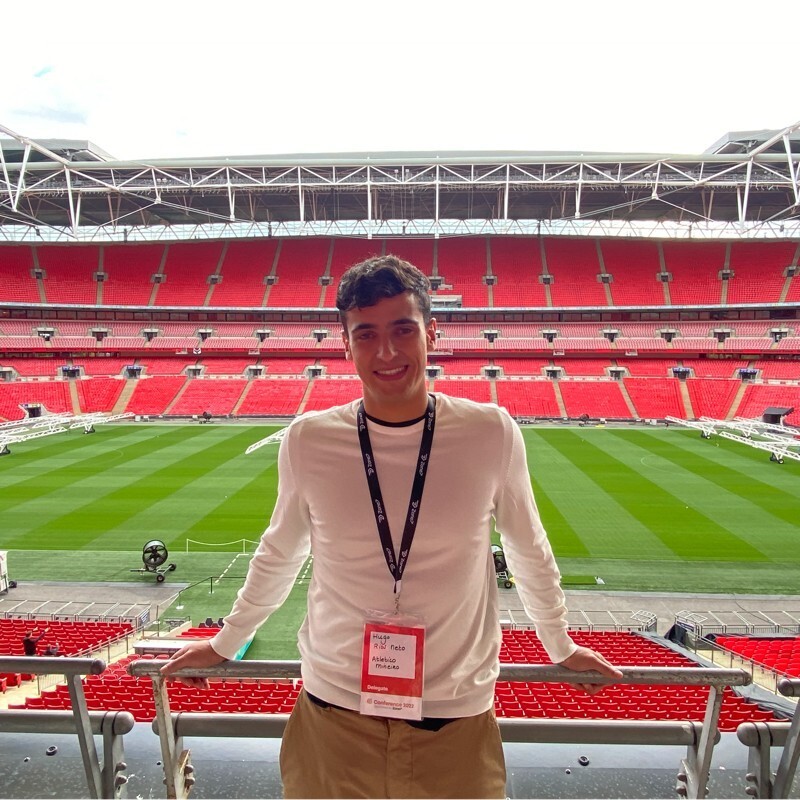
Tell us a little bit about yourself.
Certainly! I'm Hugo Rios-Neto, 23 years old, working as an Intelligence Engineer at Gemini Sports, a position I started in August 2023. Alongside my professional commitments, I'm pursuing my master’s in computer science at the Federal University of Minas Gerais (UFMG) in Brazil.
My academic and professional journeys intersect quite a bit. During my bachelor’s in computational mathematics at UFMG in 2021, I became a Data Scientist at Atlético Mineiro. I co-founded the Sports Analytics Lab (SALab) at UFMG a year later. Additionally, in a group effort between our lab at the university and the department at the club, we organized the "Football Analytics: Modeling and Experience (FAME)" event in 2022, marking it as Brazil's inaugural sports analytics event.
More recently, I had the privilege to assist in launching a new undergraduate course at UFMG, "Data Science Applied to Football," where I contributed to developing the curriculum, helping teach in almost all classes, and grading exercises. We had 60 students completing the course. Finally, I'm pleased to share that within 15 months of starting the lab with just two of us students - plus two professors, we expanded the number to eleven students.
Over the years, I've been dedicated to sports analytics, particularly in soccer. This passion has led to opportunities such as authoring a paper for the 2020 FC Barcelona Analytics Congress, co-authoring an article in the 2022 StatsBomb Conference, presenting at the 2023 Stats Perform Pro Forum, and co-authoring a paper at the Brazilian Meeting on Artificial and Computational Intelligence (ENIAC) in 2023.
What drew you to pursue an MSc?
Pursuing a master’s felt like a logical progression after my undergraduate studies, given my deep involvement in research at UFMG and Atlético Mineiro. Research plays a pivotal role in postgraduate programs in Brazil, and I was keen to immerse myself in that environment further. Moreover, I strongly desired to delve deeper into specialized areas, especially Statistical, Machine, and Deep Learning. Since UFMG has a very flexible master’s coursework structure, I only had to take one mandatory class related to fundamental algorithms, and I could take four courses in the areas mentioned above - I have just started two of them this semester. This drive was to acquire knowledge and enhance my research potential and technical proficiency.
How would you describe your time at Clube Atlético Mineiro? What role did you play on the football team?
During my tenure at Atlético Mineiro, I experienced significant personal and professional evolution. Atlético Mineiro, my hometown club, boasted the pioneering analytics department in South America. I was privileged to be a part of this department from its inception. Nurturing it from its early days taught me invaluable lessons spanning the department's multifaceted operations. I interacted with various professionals there—executive stakeholders, scouts, match analysts, goalkeeping coaches, physios, and academy coordinators. This exposure enriched my understanding of how football clubs operate and how many different areas can benefit from data analytics supporting decision-making. From a technical standpoint, my time at Atlético Mineiro marked the first instance where I engaged with the full spectrum of a data team's responsibilities. The analytics department encapsulated the entire data science lifecycle, from data engineering to dashboard creation for external stakeholders. Although my primary focus was research, particularly in model development and implementation, I played a role in always giving my opinion on the engineering and product development facets of the department. Notably, during our first year without a dedicated data engineer, I crafted a local pipeline that was scalable enough for the 30+ leagues under our purview.
What inspired you to join Gemini Sports Analytics?
Two main aspects made me join Gemini: the company's mission and my personal development. The company's mission, to be a global leader in providing world-class software as a service to sporting organizations, enhancing and maturing their systems design, architecture, and operations, is a mission I firmly believe can lead to a product that tackles many of the challenges almost all sporting organizations face. From a month in the company, the product we are building is heading precisely in that direction. Our product already supports the entire data science cycle of most sporting organizations. From a personal development perspective, it is an opportunity to try to solve problems that sporting organizations, in general, have. While the club gave me invaluable experience, it was the right time to expand my horizons and think of solutions that generalize across various teams and, ideally, sports.
With the exposure, I had at Atlético, plus talks with people who work in analytics departments at other clubs, I can translate these insights about how to tackle problems departments face into products that can directly support different components of the processes in performance departments at sporting organizations.
How do you see smaller analytics teams benefiting from the GSA app?
Sporting organizations with smaller data science teams face the very tough challenge of balancing the time dedicated to transmitting insights in the processes they are a part of with developing better systems - architecture, models, and reports - and usually have members who take care of all things data. An analytics department needs to actively participate in operations to add value in supporting decision-making, even if it has a fantastic system behind it. Developing new infrastructure, models, and reports demonstrating progress to upper stakeholders without quality systems becomes increasingly tricky.
Similarly, how do you see larger analytics teams benefiting?
Sporting organizations with larger analytics teams often have members dedicated exclusively to either technical parts of the process (data engineering, data science, and data analysis) or domain parts (scouting, match analysis, or academy). Regardless of the scenario, a team's data scientist or data analyst equipped with GSA can prototype models and dashboards very quickly, increasing the efficiency of their work, which results in a more significant number of problems they can solve within a given time frame. Regarding their data engineering needs, they could rely entirely on us to quickly ingest their data and quickly make it available in our app and API's, due to a robust cloud infrastructure.
What are you currently working on at GSA?
I am working on incorporating the VAEP framework into our product as a pre-built AI. VAEP is a framework that seeks to value individual on-ball actions players perform in Soccer based on the change in the scoring probability these actions generate. It was introduced in a paper at the 2019 KDD conference and is one of the most cited works in the Soccer Analytics literature. The metric, which values players' performance from event data - the data most clubs’ access- serves as a cornerstone to building systems for talent identification (scouting) and player development. I am very excited about implementing some of the best works developed in the Sports Analytics literature and developing new methods that can be turned into products that help drive informed decision-making from data at sporting organizations.
Are you listening to any interesting podcasts now?
I follow podcasts sparingly but rather listen to individual episodes from different podcasts that may be interesting. The Lex Fridman podcast is the one I always try to listen to when big names in the AI world are invited. Despite not being exclusively focused on AI, it is a ubiquitous topic in the podcast. Many brilliant scientists from Meta, OpenAI, Deep Mind, and other top tech companies and universities have been guests in it, detailing much of their current R&D efforts and perspectives on future directions of AI. Even though that is far from what I do on a day-to-day basis, I believe it is an excellent way of knowing what is being done at the highest level of R&D, what problems should end up being solved by these companies in the future, and to think about how translations of these approaches to sports could be like.
Where could people interested in the AI and Sports Analytics Industry learn further?
There is a vast amount of quality materials publicly available in these areas. For this question, my answer will focus on Soccer Analytics, the field I'm most familiar with. David Sumpter's Soccermatics course is a must for anyone entering the area, as it covers all the problems a data scientist will face when working for a soccer club. Another fantastic resource is KU Leuven's Sports Analytics Lab's public repositories and software, which implement many of the top papers they have published over the years. Finally, StatsBomb's courses are another excellent reference for starters.